The artificial vision uses the latest artificial intelligence technologies to provide industrial systems with the capability of seeing and analysing various activities that range from manufacturing to quality control and safety.
The artificial vision uses cameras to acquire visual information from the environment, then processes the images acquired by using a combination of hardware and software. The artificial vision technology often exploits specialized optics to acquire images, an approach that allows processing, analysing and measuring the features contained in the image with extreme precision.
In manufacturing environments, artificial vision systems are a whole of various elements: lighting, optics, sensors, frame grabbers and processors. Lighting plays the fundamental role of making the characteristics of the captured object well visible and of enhancing its main features.
The optics captures the image and transmits it to the camera sensor under the form of light source (the one reflected by the object details) to electric signals, the used technology is of CMOS (complementary metal-oxide semiconductor technology) or CCD (charge-coupled device) type.
The frame grabber, or capture card processes the image from the camera and converts it into a digital format, more precisely it acquires an analogue signal and converts it into digital output signal.
Digital signals are processed by the processor, on which specific algorithms run under the software supervision, extracting the required information from the image.
Finally, an interface connects the vision system with other elements, parts of a larger system, which might be a gantry or the automation of a manufacturing line.
An artificial vision application, as part of a manufacturing system, might be the in-line analysis of a determinate feature of an item, followed by the generation of go/no-go type information or a trend datum, to check the production stability or the tendency to go outside limits.
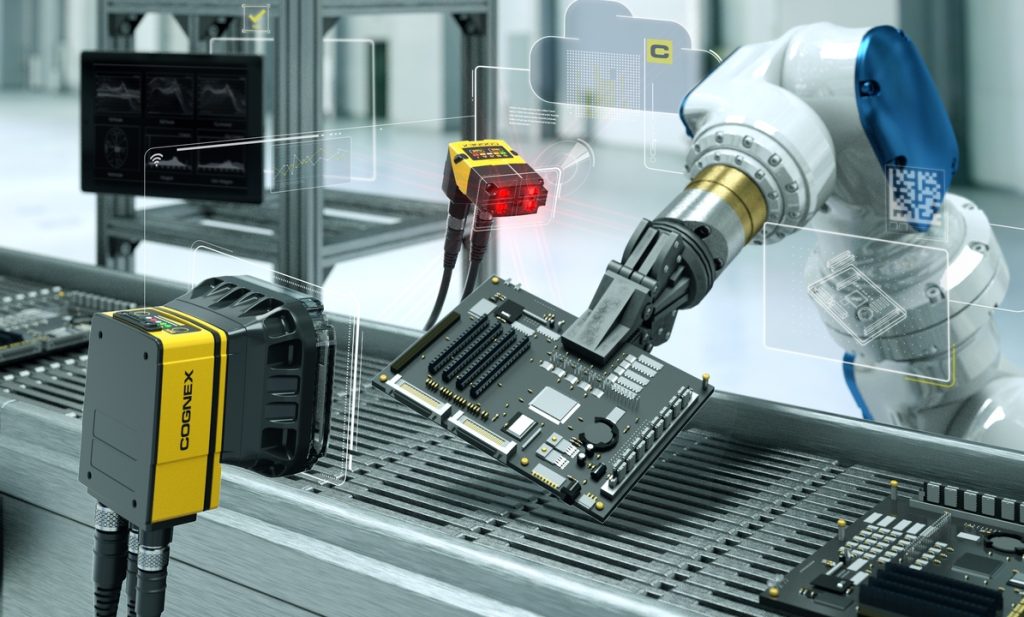
Cameras
The use of cameras is not universal, each family of cameras is specialized for a well determined task. If the use concerns for instance the area scanning, a single photogram groups more images of more objects that have the same width and height sizes. The number of pixels that characterizes the sensor corresponds to the width and to the height of the overall image.
Cameras are characterized by sensitivity and resolution. Sensitivity represents the camera capability of reproducing good-level images also according to the low-light conditions of the scene being shot.
Resolution is the measurement of the details that a camera can capture. In a digital image, it is the total number of pixels making up the image, given by the product of pixels that constitutes the base for vertical pixel one. The higher the number of pixels, the higher the resolution, and better the quality of the image and the definition of its details. In general, the higher the resolution, the narrower the field of view. Sensitivity and resolution are interdependent. If the other factors are constant, if sensitivity increases the resolution decreases, vice versa if the resolution increases the sensitivity decreases.
The use of vision systems
The applications of the artificial vision are used in a broad range of sectors, including the one of household appliances, to carry out control, analysis, calculation and tracing activities.
In the electronic production environment, the artificial vision is used on the assembly line of printed circuit boards for activities such as the solder paste inspection, the component placement and soldering results. To analyse the inspection thoroughly and to assess whether correct components are mounted, the optical character recognition (OCR) is used, as it allows the computer to extract the alphanumeric text from images.
In manufacturing ambit, there is the real-time tracing by means of barcode reading (QR code included), the recognition of models and the placement of objects, such as the positioning of a component on a whole or the one of a label on a bottle. Artificial vision functions in material inspection systems assure the quality control, with the presence of eventual defects or contaminants on a broad range of materials and products, both during and at the end of the production.
The use of cameras for the robot guide is a steeply rising area, both 2D and 3D cameras are used, exploited in both the teaching and in the operational phase. These applications provide a high investment return, decreasing the need of manpower.
In the automotive industry, the recognition of objects allows autonomous-drive cars to identify obstacles on roads.
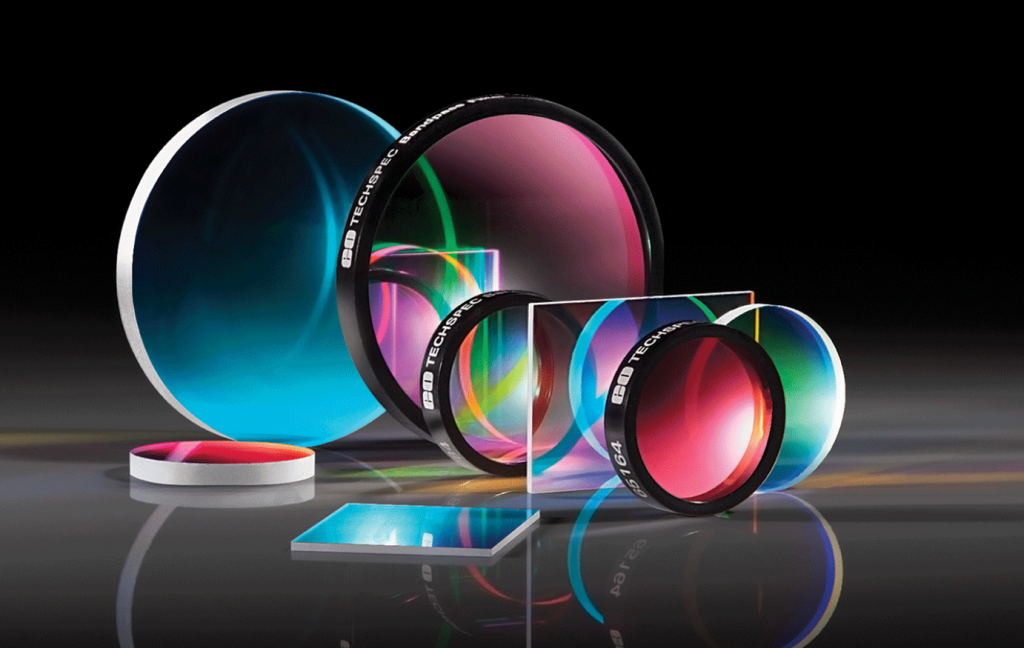
Highlights of the artificial vision
As each aspect that characterizes automation implies some advantages, the same also happens for the artificial vision, unbeatable in quantitative measurements due to its precision, coherence and speed. If integrated into a manufacturing line, for instance, a vision system can quickly inspect hundreds or even thousands of parts per minute. Using high-resolution cameras, artificial vision systems can detect and examine small details that might go unnoticed by the human eye. Moreover, the artificial vision eliminates the effects of the operator fatigue and of individual estimates, assuring objective and repeatable, in other words reliable, inspections.
By setting detection thresholds attentively, the artificial vision can identify product irregularities, such as surface dents and scratches, also distinguishing between acceptable and unacceptable defects. It can easily catch printing anomalies, such as incorrect colour shades, imperfect or missing prints.
Eventual deviations from the sample image are so promptly identified and reported for correction, granting high-quality products.
An artificial vision system can identify and measure specific points on an image, such as diameter, radii of curvature, distance and depth. It becomes an aid to determine, for instance, the inner diameter of a hole in a motor or the filling level of a liquid in a container.
It shortens idle times because it does not need pauses and has no mechanical gears in motion, then no wear and costs linked with repairs. Concerning costs, an artificial vision system can increase the manufacturing speed and decrease the manpower needed to make production work. It can also minimize the reject rate, by reducing the waste of materials. Last but not least, it improves the work safety by minimizing the need of human intervention during the manufacturing process and also the contact with dangerous parts or materials may be limited.
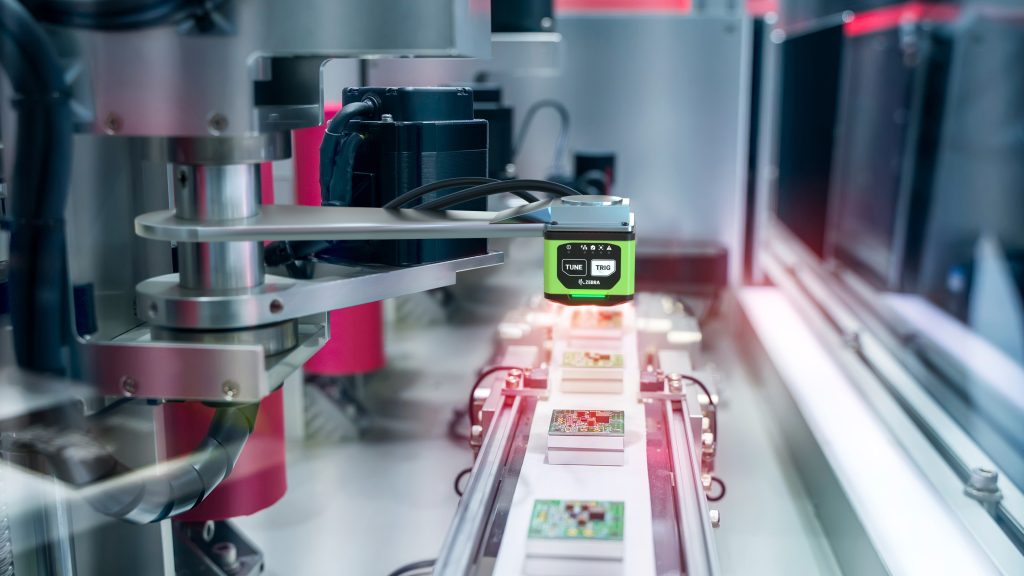
Vision and artificial intelligence
The artificial intelligence can process a large number of images and information; therefore, it is used in vision processes to speed up the decisional development.
In the manufacturing industry, the artificial intelligence aids in the recognition of objects and in the inspection of materials, to allow artificial vision systems to understand acceptable variations in the shape and in the structure of an object or of a material.
In the quality assurance, it helps interpret anomalies, without rejecting all that does not strictly mirror a specification.
The theme is enlarged to deep learning techniques that extend the artificial intelligence to those industrial applications where it is necessary to take decisions of extreme precision. When, for instance, there is the presence of dust in an image or when it is necessary to distinguish among extremely similar elements. The deep learning uses artificial neural nets organized in layers, where each layer calculates the values for the successive one, which allows processing the information in more and more complete and in-depth manner.
Neural networks allow processing enormous quantities of data, leading the association with the deep learning to represent an alternative to the traditional artificial vision.
Today the vision needs a high-speed real-time processing with low latency, this results in the use of solutions based on the Edge Computing.
The network latency is the delay in communication, it measures the time taken for data to travel across the network. Networks with long delay have high latency, whereas those with quick response times have a low latency.
Hence the use of the edge computing, a form of data processing that is executed locally, close to their origin, therefore decreasing the need of processing them in a remote data centre, then sending smaller-size packages (with minor latency incidence).
Due to the possibility to transferring data to cloud more quickly, companies are starting exploiting its potentialities, not only for filing and sharing, but especially for the analysis and the use of algorithms of artificial intelligence and deep learning.